A common mistake I make when experimenting with AI is treating it like a question-answering machine.
Google has trained us to think this way. You type in a query, and it hands you a trail of crumbs pointing in roughly the right direction. You search, browse, and assemble the puzzle pieces yourself. It's an ingrained habit.
AI, of course, does a surprisingly good job of this too. If you ask Perplexity or ChatGPT something like "What is the history of tariffs and their economic effects?" you'll get a detailed, coherent answer almost instantly. Google will give you that too, eventually—though after you've sifted through multiple tabs. The end result, though, feels quite similar: you have a question, and you receive an answer.
But if that's all you ever do with AI, you'll soon hit some limits. The responses can feel vague, overly general, even occasionally wrong. You might find yourself underwhelmed.
Tyler Cowen explains this behaviour well:
"They're asking it questions that are too general and not willing enough to give it context, so they end up insufficiently impressed by the AI. But if you keep on whacking it with queries and facts and questions and interpretations, you'll come away much more impressed than if you just ask it simple questions. At the end of the day, if you do that, you'll be asleep on the revolution occurring before our eyes: AI is getting smarter than we are. You'll just think it's a cheap way to achieve a lot of mid-level tasks, which it also is."
So if AI isn't just an answering machine, then what exactly is it?
Bring in the Army
A better way to think about AI is to imagine you have an infinite army of smart, eager interns.
They're enthusiastic but require direction.
Give them vague instructions and you'll receive vague results. You wouldn't send a one-line email saying, "Figure this out" and expect great outputs. Instead, you'd carefully explain the task, detail precisely what you're looking for, and provide useful reference materials.
In other words, it's less about getting answers and more about enhancing the quality and speed of your own thinking, leveraging your own knowledge and context. This starts with proper prompting, but its more nuanced than that. If you use ChatGPT o1 or DeepSeek you can observe the chain of thought reasoning as it processes in real time. You can watch the model think and process your query as it compiles a response. What you'll notice is that at the heart of great prompt is great context. Its hard to prompt AI's with what we don't know. The more you bring to the party, the better the answers will be because you can spot the errors and fine tune.
Expert In The Loop
Our brains excel at making relative judgments, not absolute ones. It’s easier to say “this is better than that” than to assign an objective score. This is why expert taste, in any field, is built on comparisons, not formulas.
(Its hard to describe how tall someone is. But its a lot easier to look at two people and say who is taller. Its clearer)
Taste isn’t built on checklists. It’s built on contrast. The best writers, the best designers, the best decision-makers—they don’t just know what’s “good.” They know what’s better.
That "skill" we are poking at is defined as tacit knowledge. Tacit knowledge are the insights people gain naturally through experiences, interactions, and observations without consciously realizing it.
AI can write an essay, but it doesn’t know what makes a great one—it just mimics patterns. If AI is good at formulas and automation, then human advantage comes from judgment, intuition, and taste—things learned over time.
An expert chef doesn’t measure every spice with precision; they sense what the dish needs. An AI can suggest a recipe, but it won’t invent a new one the way a seasoned chef will. Pattern matching, or reverse pattern matching, is foundational to expertise.
A radiologist will interpret a x-ray a lot better than a lay person, but can leverage the rapid and comprehensive knowledge of an AI tool to speed up the process.
Having an expert-in-the-loop, applying tacit knowledge and experience, will always create a better result.
Here is another example. When hiring a top law firm, you're paying for more than precedents and intellectual horsepower; you're paying for the nuanced judgment and deep experience of the partner managing your file. Large language models can handle the first parts efficiently, but the essential human expertise is the secret sauce. Tacit knowledge is what allows the process to function at its best.
Here is the interesting thing. Tacit expertise makes humans valuable in an AI world as the "expert in the loop"
Here is how this looks at a high level:
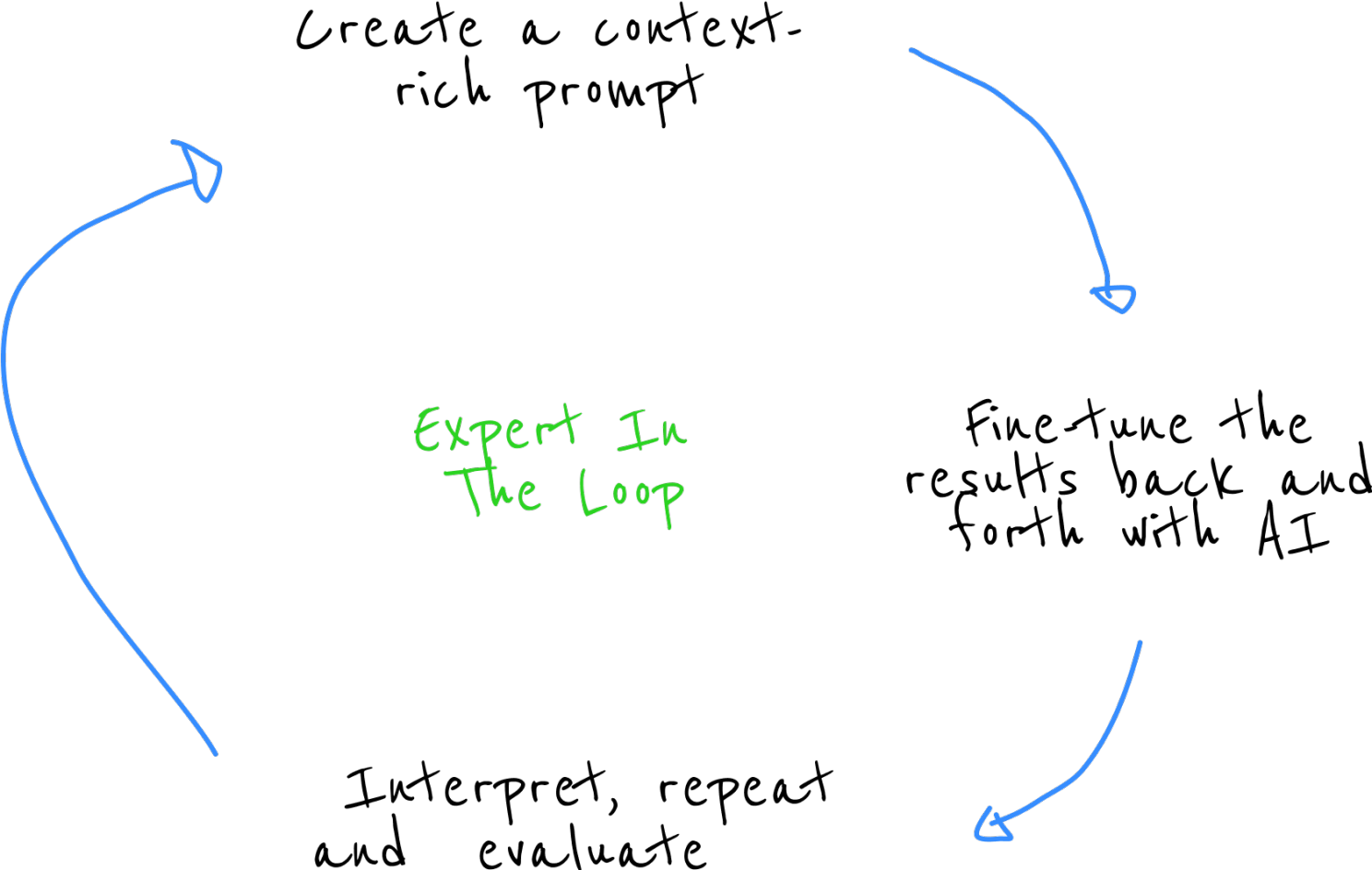
Leverage, not Answers
AI will keep getting better at the explicit things, the measurable things, the problems we’ve already defined. But the the intuitive leaps, the gut-feelings will remain uniquely human.
The best results are found when you really push into the details and continuing to refine the answer set. One thing that I have found to be surprisingly helpful in delivering higher quality responses is using phrases like "Think Hard" and "Think Deep and Longer" and requesting revisions when I think the quality isn't up to snuff. For some reason this is hard to remember to do! It isn't a normal pattern if you are used to using search engines for discovery and learning.
The future won’t belong to those who treat AI like Google, asking questions and hoping for answers. It’ll belong to those who learn to direct this infinite army of interns and pair AI’s tireless pattern-matching with their own taste and judgment.
In other words: don’t outsource your intuition. Leverage it.